Researchers at the University of Barcelona are investigating new machine learning tools to ensure millions of elderly adults can obtain a timely diagnosis of a serious yet largely under-diagnosed heart condition.
The risks of Atrial Fibrillation (AF)
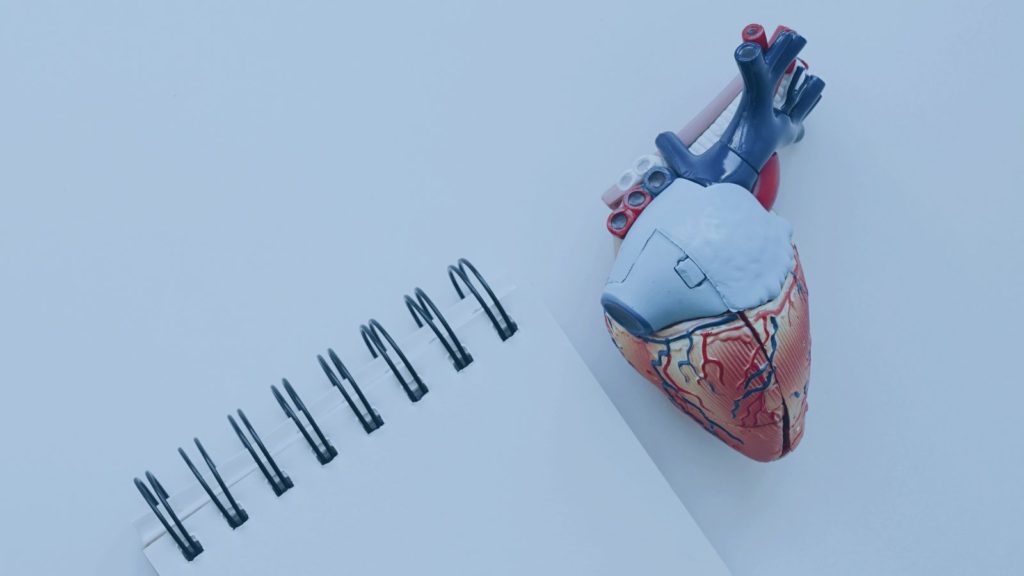
Atrial fibrillation (AF) affects more than 14 million people over the age of 65 and causes a five-fold increased risk of stroke, doubles the risk of myocardial infraction, and results in a greater risk of heart failure and death, leading to the European Society of Cardiology to declare it a condition that requires urgent action.
To improve the detection of AF, the University of Barcelona conducted a study entitled ‘Functional requirement analysis report of Atrial Fibrillation use case’. This study analyses the UB’s proposed technique for improving the detection of AF by evaluating the legal, ethical, and data requirements needed to do so. Given the asymptomatic nature of AF at an early stage, detection often only occurs when a patient experiences at late stages when the patient has severe symptoms or suffers from a stroke. Researchers believe that early detection could help prevent stroke through the personalised prescription of anticoagulation treatments and improved monitoring overall.
Machine learning techniques
Various machine learning techniques are increasingly being integrated into the health sector to help combine and analyse relevant data to improve the diagnosis, prevention, and treatment of a variety of conditions. In the case of AF, researchers employed an unsupervised machine learning technique to identify atrial fibrillation phenotypes and risk factors that are said to predispose AF. A group of AF sufferers from the UK Biobank (UKBB) was examined to help identify the requirements needed for the successful application of the technique, including its administrative, legal, and ethical requirements.
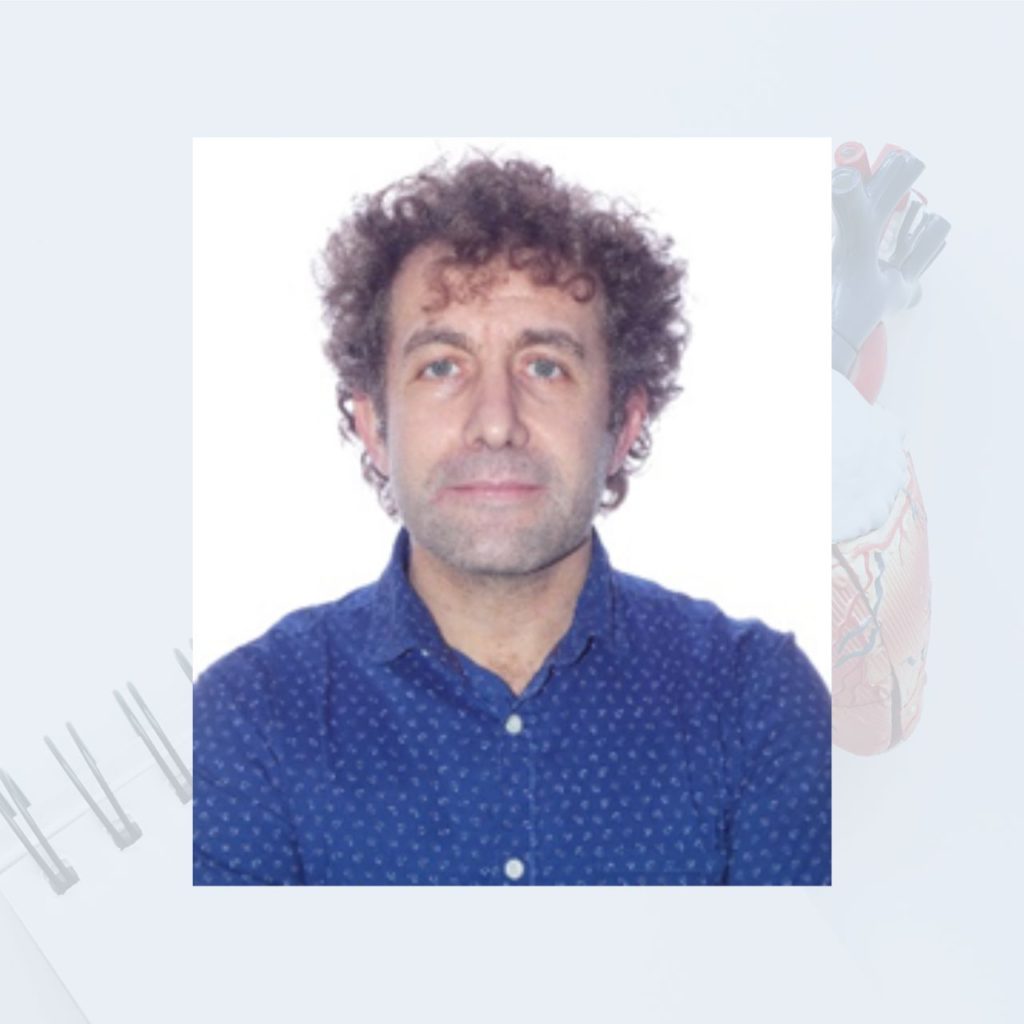
Data-driven quantification in atrial fibrillation has been explored and defined as a guiding use case for HealthyCloud to address legal requirements, data discoverability, data access and federated analytics
Karim Lekadir, lead researcher
The data analysed included health clinical data (medical and family history), biomarkers, genetic variants, imaging, ECGs, lifestyle information, and data from research studies. The study was then extended to other AF groups to generalise the model in a federated learning scheme. This exercise helps to inform what should be included in the design of future analyses. Study leader, Karim Lekadir, emphasizes that ‘data-driven quantification in atrial fibrillation has been explored and defined as a guiding use case for HealthyCloud to address legal requirements, data discoverability, data access and federated analytics.
Data challenges
This study revealed important challenges for AF modelling. First, there was limited data on AF events, which means there is a need for multi-centre approaches. Data from different groups raised the issue of heterogeneity and imbalanced data. The study also details the steps required for requesting and receiving data in both centralised and federated systems and revealed the data access challenges when requesting the data. A federated system, which the study identifies as the ideal scenario, was seen as being especially complex due to its additional hardware requirements as the training is done locally for each centre.
This study represents one of the two use cases of the HealthyCloud project, which seeks to identify the functional requirements of two real-world research questions whose answers require linking health research data, clinical data and administrative data. It contributes to the project’s objective of providing recommendations, guidelines and best practices for accessing, using and reusing health data for better research outcomes across Europe.
For more information on this study, see the full report here.